Due to the highly complex nature of both human and physical systems, the ability to comprehend them and model future conditions using a watershed approach has taken a geographic dimension. Satellite remote sensing and Geographic Information Systems (GIS) technology have played a critical role in all aspects of watershed management, from assessing watershed conditions through modeling impacts of human activities to visualizing impacts of alternative scenarios (Tim & Mallavaram, 2003).
The extreme weather phenomena and global warming noted in recent years has demonstrated the necessity for effective flood risk management models. According to this paradigm, a considerable shift has been observed from structural defense against floods to a more comprehensive approach, including appropriate land use, agricultural and forest practices (Alexakis et al., 2013a, 2013b; Barredo & Engelen, 2010; Lilesand & Kiefer, 2010; Michaelides et al., 2009). Land cover changes may be used to describe the dynamics of urban settlements and vegetation patterns as important indicators of urban ecological environments (Yinxin & Linlin, 2010). Satellite remote sensing provides an excellent source of data from which updated land use / land cover (LULC) changes can be extracted and analysed in an efficient way. In addition, effective monitoring and simulating of the urban sprawl phenomenon and its effects on land-use patterns and hydrological processes within the spatial limits of a watershed are essential for effective land-use and water resource planning and management (Hongga et al., 2010; Hadjimitsis et al., 2004a, 2010a, 2010b). Several techniques have been reported in order to improve classification results in terms of land use discrimination and accuracy of resulting classes in the processing of remotely sensed data (Agapiou et al., 2011). As a result of Very High Resolution (VHR) imagery, real world objects that were previously represented by very few pixels, are now represented by many pixels. Thus, techniques that take into account the spatial properties of an image region need to be developed and applied. One such technique is texture analysis (Zhang & Zhu, 2011). Moreover, during the last years, spatial metrics have been largely used in landscape studies. According to Haralick et al. (1973), landscape metrics capture the inherent spatial structure of the environment and are used to enhance interpretation of spatial pattern of the landscape.
Several techniques have been reported to improve classification results in terms of land use discrimination and accuracy of resulting classes (Eiumnoh & Shrestha, 2000). However, the multispectral images acquired from different satellite sensors suffer from serious problems and errors, such as radiometric distortions, areas with low illumination, physical changes of the environment, etc. Recent studies have found that the accuracy of classification of remote sensing imagery does not increase by improving the applied algorithms, since classification mainly depends upon the physical and chemical parameters of the objects on the ground (Rongqun & Daolin, 2011).
Soil erosion is considered to be a major environmental problem, as it seriously threatens natural resources, agriculture and the environment in a catchment area. Spatial and quantitative information of soil erosion contributes significantly to the soil conservation management, erosion control and general catchment area management (Prasannakumar et al., 2011). In recent years, there has been a growing awareness of the importance of problems directly related to erosion in the broader Mediterranean region. The widespread occurrence and importance of accelerated erosion in the Mediterranean region has driven to the development of models at scales ranging from individual farm fields to vast catchment areas and different types of administrative areas (Bou Kheir et al., 2008). In some parts of the Mediterranean region, erosion has reached a stage of irreversibility, while in some places there is no more soil left (Kouli et al., 2009). Although soil erosion is characterized as a natural phenomenon, human activities such as agriculture can accelerate it further (Karydas et al., 2009).
Recently, space-born microwave active remote sensing, especially Synthetic Aperture Radar (SAR) with its all-weather capability, can provide useful spatially distributed flood information that may be integrated with flood predictive models in the construction of an effective watershed management. Radar imagery is useful for the identification, mapping and measurement of streams, lakes and inundated areas. Most surface water features are detectable on radar imagery due to the contrast between the smooth water surface and the rough land surface (Lewis, 1998). The amount of moisture stored in the upper soil layer changes the dielectric constant of the material and thus affects the SAR return. Because the dielectric constant of water is at least 10 times bigger than that of the dry soil, the presence of water in the top few centimeters of bare soil can easily be detected through the use of SAR imagery (Lillesand & Kiefer, 2000). In addition, the differences in the values between the dielectric constant of water and of dry soil at the microwave part of the spectrum plays a major role in the soil moisture estimation through the use of microwaves.
The main aim of this chapter is to integrate all the individual remote sensing methodologies related to watershed monitoring and management in a holistic approach. Specifically, different approaches such as development of erosion models, use of radar imagery for the detection of areas prone to inundation phenomena, construction of Land Use /Land Cover (LULC) maps, optimization of classification methodologies and calculation of landscape metrics for the recording of urban sprawl will be presented thoroughly and will highlight the contribution of satellite remote sensing to the sustainable management of a catchment area.
Study area
Located in the central part of the island of Cyprus, the Yialias basin is about 110 km2 in size (Fig. 1). This study area is situated between longitudes 33°11´24.28´´ and 33°26´31.52´´ and latitudes 34°54´36.74´´ and 35°2´52.16´´. Cyprus is located in the Northeastern corner of the Mediterranean Sea and, therefore, has a typical eastern Mediterranean climate: the combined temperature–rainfall regime is characterized by cool-to-mild wet winters and warm-to-hot dry summers (see Michaelides et al., 2009).
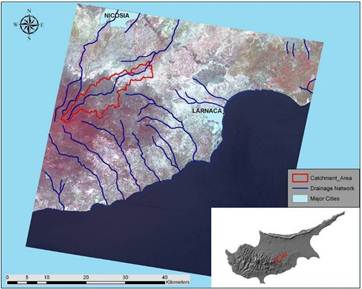
FIGURE 1.
The study area
Development of methodology for the optimization of classification accuracy of Landsat TM/ETM+ imagery in a catchment area in Cyprus
INTRODUCTION
An important tool for the detection and quantification of land cover changes across catchment areas is the classification of multispectral satellite imagery, as such results are very important for hydrological analysis and flood scenarios.
This study aimed at testing different material samples in the Yialias region (central Cyprus) in order to examine: a) their spectral behavior under different precipitation rates and b) to introduce an alternative methodology to optimize the classification results derived from single satellite imagery with the combined use of satellite, spectroradiometric and precipitation data.
DATA AND METHODOLOGY
GROUND SAMPLE
According to preliminary classification results (Alexakis et al., 2011), spectral mixing between urban areas and specific geological formations was observed. Thus, samples of regolith and construction material were collected and tested for their spectral response under different conditions of humidity with the use of spectroradiometer in the premises of the Remote Sensing and Geomatics Laboratory of Cyprus University of Technology (Alexakis et al., 2012).
SATELLITE AND PRECIPITATION DATA
For the purposes of the study, specific tools and data were incorporated:
· Four Landsat TM/ ETM+ multispectral images of medium resolution (30×30 m2 pixel size).
· Precipitation data obtained from the Meteorological Service of Cyprus (Pera Chorio Meteorological Station : Lon – 35° 01’, Latitude – 33° 23’). All of these data were compared with the satellite imagery data. Selected satellite imagery was retrieved a day after the recording of substantial scaling amount of precipitation from the Pera-Chorio Metereological Station.
· Data derived from spectroradiometric field campaigns. For this reason a GER 1500 spectroradiometer was used. This instrument can record electromagnetic radiation between 350 nm up to 1050 nm (Fig. 2).
In order to investigate the different spectral response of each sample under different moisture conditions, all samples were immersed in water in a step-by-step process and measured for the rate of their humidity with a soil moisture meter. The specific hand-held instrument used in this study was able to measure moisture values from 0 to 50% within an accuracy of 0.1%. The final under investigation regolith samples were divided in four different categories, according to their level of humidity: 0% (dry sample); 25%; 50%; > 50%. With regard to tile and roof specimens, the results were divided into “dry” or “humid” categories due to the difficulty to measure the scaling levels of humidity in those kinds of materials.
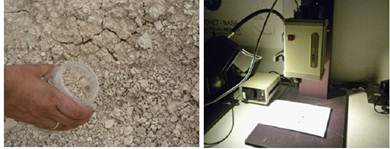
FIGURE 2.
Collection of soil data (left). Spectroradiometric measurements of material samples at the premises of the Remote Sensing and Geomatics Laboratory of CUT (right)
Based on the results of the scatter-plots, it was found that in the case of dry samples there is a strong spectral confusion between the chalk A response and the urban fabric (roof and tile) materials. The “moisture” scatter plot (humidity > 50%) highlights the different spectral response between artificial materials (roof and tile) and natural materials (chalk A, B, C). In this plot, the spectral difference between different samples is increased and two major clusters are created with complete contrary spectral response (increase of chalk A spectral response and substantial decrease of tile and house roof -constructed from clay and cement consecutively- spectral response, see Fig.3).
The results highlighted the different spectral response of materials under different humidity levels. Specifically, reflectance values of chalk samples (samples A and C) tend to be separated from those of urban samples (tile and roof) as humidity increases.
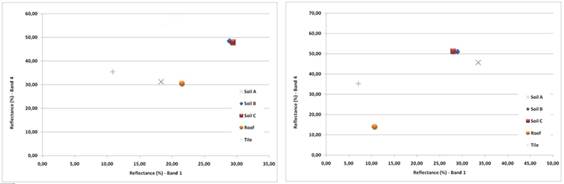
FIGURE 3.
Scatter-plots of the different targets examined in this study for Band 1 – Band 4 (humidity 0%) (left) and Band 1 – Band 4 of Landsat (humidity > 50%) (right)
SATELLITE IMAGERY DATA
After the application of all necessary pre-processing steps (radiometric, atmospheric and geometric corrections,) spectral signature profiles were extracted for all of the different materials during the acquisition dates of each satellite imagery (Fig. 4).
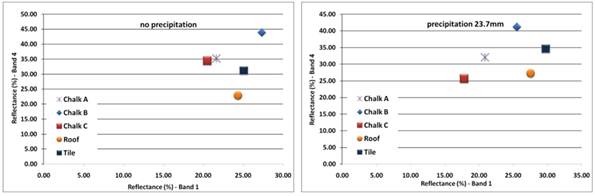
FIGURE 4.
Scatter-plots of the different targets examined in this study for Band 1 – Band 4 (left) and Band 3 – Band 4 of Landsat (right)
The results of the scatter plots denoted the scaling optimization of spectral separability of satellite imagery data, from 0 to 23.7 mm of precipitation. Specifically, concerning the 0 mm precipitation case, a spectral confusion was indicated between the “urban” targets (roof and tile) and chalk A and C targets. This conflict was outreached gradually as the precipitation level increased. The samples started to have different spectral behaviour, with the chalk samples (except chalk B) standing gradually away from the “urban” samples cluster in the scatter-plot. It is important to mention the quite different spectral response of chalk C sample in satellite images compared to its response in the laboratory specimens. This problem occurred due to the medium spatial resolution of Landsat images (30×30 m2 pixel size) which increases the likelihood of the common mixing pixel phenomenon.
RESULTS AND VERIFICATION
The results from the laboratory and satellite imagery analysis methods highlighted the different spectral response of materials to different levels of humidity. For the direct comparison of the classification accuracy between images, where different levels of precipitation have been recorded, two Landsat TM/ETM+ images acquired on 2 June 2005 (0 mm precipitation – “dry”) and 23 July 2009 (23.7 mm precipitation – “rainy”) were classified and compared (Fig. 5). Both unsupervised (ISODATA) and supervised classification algorithms (Maximum Likelihood – ML) were used. Initially, the ISODATA classification technique was applied to both images with 95% convergence threshold. The following 5 classes were used for both the supervised and unsupervised algorithms: 1) urban Fabric, 2) marl – chalk formations, 3) vegetation, 4) bare soil and 5) forest.
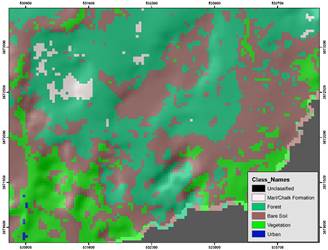
FIGURE 5.
Detail of the “rainy” satellite image after the application of supervised classification algorithm
On the one hand, the results of the unsupervised algorithm performance for both dry and humid acquisition days could be described as poor and were not considered for further evaluation (Kappa coefficient of classification accuracy – (Kc) < 60%). On the other hand, the application of supervised algorithm to “rainy” image provided better accuracy results (Kc = 0.75). The product of “dry” image was substantially better than that of unsupervised case but with insufficient accuracy to be considered as credential.
CONCLUSIONS
The results noted the importance of imagery acquisition date for optimization of classification results. Specifically, the overall accuracy of classification product was substantially increased (more than 30% for supervised classification), especially for urban and marl/chalk areas, during days where high precipitation measurements were recorded in the broader study area. The results were established either by laboratory or satellite imagery analysis.
Assessing soil erosion rate in a catchment area in Cyprus using remote sensing and GIS techniques
INTRODUCTION
The objective of this work was to develop and evaluate two different erosion models in the catchment area of Yialias in Cyprus. The first was an empirical multi-parametric model which is mainly based on expert’s knowledge (Analytical Hierarchical Process – AHP) and the second (Revised Universal Soil Loss Equation – RUSLE) was the model which is considered to be a contemporary simple and widely used approach of soil loss assessment.
METHODOLOGY
RUSLE METHODOLOGY
The RUSLE equation incorporates five different factors concerning rainfall (R), soil erodibility (K), slope length and steepness (L and S. respectively), support practice (P) and cover management (C):
A=R∗K∗L∗S∗P∗CA=R∗K∗L∗S∗P∗C | (1) |
AHP allows interdependences between decision factors to be taken into account and uses expert opinions as inputs for evaluating decision factors. The final weight of significance for each factor can be defined by using the eigen-vectors of a square reciprocal matrix of pairwise comparisons between the different factors. Moreover, a specific grade is assigned to all the different pairs from 1/9, when the factor is “not important at all”, to 9, when the factor is “extremely important”.
RAINFALL (R) FACTOR
The rainfall factor R is a measure of the erosive force of a specific rainfall value. For the calculation of the R factor with the use of the Modified Fournier Index (MFI), the following two different approaches suggested by Ferro et al. (1991) and Renard & Freimund (1994) for the areas of Sicily and Morocco were used respectively :


According to Kouli et al. (2009), MFI is well correlated with the rainfall erosivity. The specific index is considered as an effective estimator of R because it takes into account the rainfall seasonal distribution. Therefore the MFI was applied to take into account the monthly rainfall distribution during each year for a period of 20 years, as follows:

where, Ff is the MFI index, pij is the rainfall depth in month / (mm) of the year j and P is the rainfall total for the same year. After the calculation of R, a continuous surface was produced using the ordinary Kriging method based on Gaussian function, which was found to be the most effective for the production of the final iso-erosivity map. The mean values of R range from 267 MJ mm ha year-1 in the most flat areas in Yialias watershed to 694 MJ mm ha year-1 in the mountainous and generally steep areas.
SOIL ERODIBILITY (K)
The soil erodibility factor (K) refers to the average long-term soil and soil profile response to the erosive power associated with rainfall and runoff. It is also considered to represent the rate of soil loss per unit of rainfall erosion index for a specific soil.
A digital soil map of the study area was used and the main soil formations were categorized in three different major classes: coarse sandy loam, sandy loam and silty clay. According to Prasannakumar et al. (2011) the estimated K values for the textural groups vary from 0.07 t ha h ha-1 MJ-1 mm-1 for coarse sandy loam, 0.13 t ha h ha-1 MJ-1 mm-1 for sandy loam and 0.26 t ha h ha-1 MJ-1 mm-1 for silty clay.
TOPOGRAPHIC FACTOR (LS)
The topographic factor is related to the slope steepness factor (S) and slope length factor (L) and is considered to be a crucial factor for the quantification of erosion due to surface run–off.
The combined topograpfic factor was calculated by means of ArcGIS spatial analyst and Hydrotools extension tools. In this study, the equation derived from Moore & Burch (1986) has been adoped:

PRACTICE FACTOR (P)
The practice factor (P) is defined as the ratio of soil loss after a specific support practice to the corresponding soil loss after up and down cultivation. In order to delineate areas with terracing practices, the two GeoEye-1 satellite images were used and the delineation was accomplished in GIS environment with extensive monitoring of the study area. Areas with no support practice were assigned with a P factor equal to 1. However, the terrace areas which are considered to be less prone to erosion were assigned a 0.55 value, according to expert’s opinion.
COVER MANAGEMENT FACTOR (C)
According to Prasannakumar et al. (2011), the C factor represents the effect of soil-disturbing activities, plants, crop sequence and productivity level, soil cover and subsurface bio-mass on soil erosion.
The NDVI (Normalised Difference Vegetation Index) extracted from the study area (applied to GeoEye-1 image) has values that range from -0.65 to 0.99. The NDVI is used along with the Equation 6 in order to calculate the C factor values of the study area in GIS environment.

where, a and b are non-dimensional parameters that determine the shape of the curve relating to NDVI and C factor.
According to the final results, C factor values ranged from 0 to 2.7.
APPLICATION OF RUSLE METHODOLOGY FOR SOIL LOSS ESTIMATION
The annual soil loss was calculated in a GIS environment (Fig. 6), according to Eq. 1. According to the final results, the estimated soil loss ranges from 0 to 6394 t ha-1 yr-1 with a mean value of 20.95 t ha-1yr-1. The maximum value of 6394 t ha-1 yr-1 cannot be considered as appreciable due to the fact that only one pixel in a total of 1199 was attributed with this value. However, the mean value of 20.95 t ha-1yr-1 is representative of the current soil loss regime of the basin.
AHP METHODOLOGY
In the AHP methodology, interdependencies and feedback between the factors were considered. The factors used in this methodology were: rainfall (R), soil erodibility (K), slope length and steepness (LS), cover management (C), support practice (P) and stream proximity. Six out of seven factors had already been analyzed in the RUSLE methodology. The additional agent to be analyzed was the proximity to rivers and streams.
PROXIMITY TO RIVERS AND STREAMS
According to Nekhay et al. (2009), an area of 50 m around rivers and streams was considered to be prone to flooding and, consequently, to the detachment of particles of soil by floodwaters. Thus, initially with the use of ArcGIS 10 Hydrotools module, the drainage network of the basin was automatically extracted from the hydrological corrected DEM (Digital Elevation Model). Next, a buffer zone of 50m was constructed around each drainage network segment.
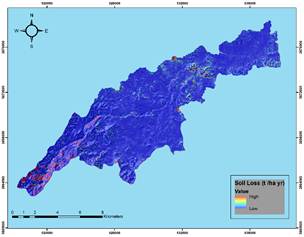
FIGURE 6.
Map of the spatial distribution of soil loss after the application of RUSLE methodology in Yialias catchment area
According to AHP methodology, a pair-wise comparison of the contribution of each factor was established. Specifically, answers of several experts were collected on the reciprocal matrix, and the appropriate eigenvector solution method is then employed to calculate the factor weights.
The final soil erosion risk map (Fig. 7) was constructed by summing up (through Boolean operators) the product of each category (that had already been rated accordingly for its subcategories) with the corresponding weight of significance according to the following equation:

Where F1, F2,…, FN are the different factors incorporated in the model.
The final erosion risk assessment map was reclassified to three soil erosion severity classes separated as low (pixel value 1), moderate (pixel value 2) and high risk (pixel value 3). The results denoted that 77.5% of the study area was classified as low potential erosion risk, 17.5% as moderate potential risk and only a 5% as high risk.
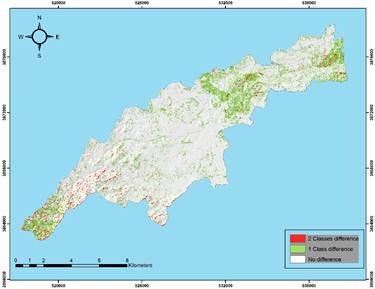
FIGURE 7.
Final erosion risk map constructed with AHP method
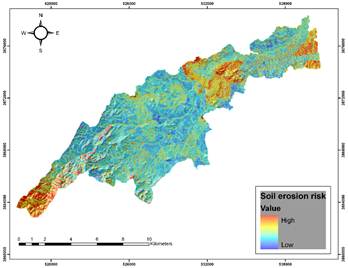
FIGURE 8.
Image indicating the soil erosion severity class differences between AHP and RUSLE method
EVALUATION OF AHP AND RUSLE
In the same way that the AHP risk assessment map was reclassified, the estimated soil loss percentage map was separated in 3 different classes according to experts opinion (1st class 0-20 t ha-1 yr-1 for pixel value 1, 2nd class 20-100 t ha-1 yr-1 for pixel value 2, 3rd class 100-6391 t ha-1 yr-1 for pixel value 3). The two grid images were subtracted in GIS environment. A close look at the extracted grid image, it is obvious that there is a considerable similarity between the two methodologies (Fig. 8).
CONCLUSIONS
This research demonstrated the potential for the integration of RS, GIS and precipitation data to model soil erosion. The current research found that both RUSLE and AHP methodologies can be efficiently applied at a basin scale with quite modest data requirements in a Mediterranean environment such as Cyprus, providing the end users with reliable quantitative and spatial information concerning soil loss and erosion risk in general.
Flood mapping of Yialias river catchment area in Cyprus using ALOS PALSAR radar images
INTRODUCTION
ALOS (Advanced Land Observing Satellite) PALSAR data can be used to detect the water surface due to the L-band wave length. All SAR instruments share the advantages of day-night operability (as active sensors), cloud penetration, and the ability to calibrate without performing atmospheric corrections. The longer L-band (~23.5 cm) SAR wavelength, and, to a certain extent, the C-band (~5.5 cm), have the ability to penetrate vegetation canopies to various degrees depending on vegetation density and height, dielectric constant (primarily a function of water content), and SAR incidence angle. Variations in backscattering allow discrimination among non-vegetated areas (very low to low returns), herbaceous vegetation (low to moderate returns), and forest (moderate to high returns), and to some degree among different forest structures and regrowth stages. Where water is present beneath a forest canopy, enhanced returns caused by specular “double bounce” scattering between water surface and tree trunks makes it possible to distinguish between flooded and non-flooded forest.
DATA AND METHODOLOGY
DATA AND METHODOLOGY
The purpose of this study is to explore the potential of ALOS-PALSAR imagery for observing flood inundation phenomena in the Yialias catchment area in Cyprus. Two PALSAR images (polarity: HH, pixel size 50 m) covering the study area before and after an extreme precipitation incident in 2009 were used (Table 1). A LULC map was also constructed with the use of high resolution images such as GeoEye -1 covering the study area. To analyze Radar backscatter behavior for different land cover types, several regions of interest were selected based on the land cover classes. A number of land cover classes were found to be sensitive to flooding, whereas in some other classes backscatter signatures remained almost unchanged.
DATA
For the purposes of the study, the following satellite and digital spatial data were incorporated:
· 2 GeoEye -1 images
· A Digital Elevation Model (DEM) of 25m pixel size provided by the Department of Land and Surveys of Cyprus, created with the use of orthorectified stereopairs of aerial photos covering the study area.
The ALOS images were acquired on 30 November 2009 and 6 December 2009 (Fig. 9a). PALSAR is a fully polarimetric instrument, operating at L-Band with 1270 MHz (23.6 cm) centre frequency and 28 MHz, alternatively 14 MHz, bandwidth. The antenna consists of 80 transmit /receive (T/R) modules on four panel segments, with a total size of 3.1 by 8.9m (Table 1). The two ALOS images were acquired after thorough indexing of Cyprus Meteorological Service archives of precipitation data. Specifically, the research team searched the precipitation archives of all the meteorological and climatological gauge stations within the study area (Analiontas, Pera Chorio, Lythrodontas, Mantra tou Kampiou, Kionia, Mathiatis), as they are indicated and spatially distributed in Fig. 10. Due to the lack of ALOS imagery data acquired during recorded flood inundation events, the research team tried to acquire images before and after extreme precipitation events in order to examine the potential of the imagery to detect soil moisture and flood inundation trends. Thus, the image for 30 November 2009 corresponded to a day where no precipitation had been recorded, while the image for 6 December 2009 corresponded to a day when a mean value of 25mm of precipitation had been recorded in the rain gauge stations within the study area.
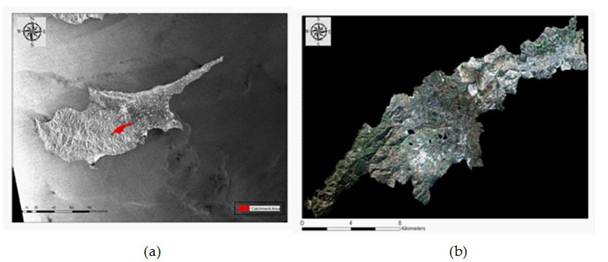
FIGURE 9.
(a) ALOS PALSAR image (30 November 2009) and the study area. (b) Mosaic of the two GeoEye -1 images of the study area (RGB – 321)
The GeoEye-1 images were used for the land use monitoring of the upstream and downstream of the basin; the two images refer to 12 March 2011 and 11 December 2011, respectively. GeoEye-1 is a multispectral sensor with four spectral bands. Its spectral range is: 450-510 nm (blue), 510-580 nm (green), 655-690 nm (red) and 780-920 nm (near infrared), while its spatial resolution is approximately 1.65 m.
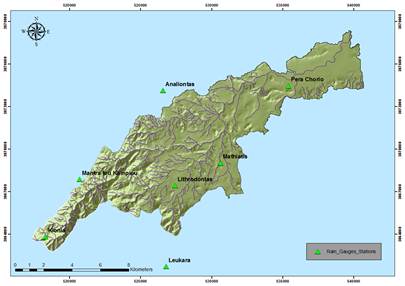
FIGURE 10.
Rain gauge stations within the study area or in close vicinity with it and drainage network
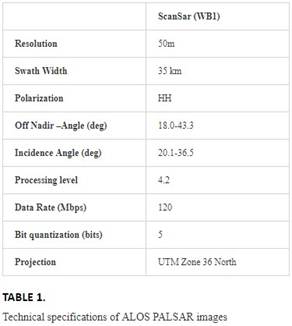
PRE-PROCESSING TECHNIQUES
Initially, geometric corrections were carried out to ALOS PALSAR images using standard techniques with ground control points and a first order polynomial fit so asthe two images to be co-registered. For this purpose, topographical maps were used to track the position of ground control points in conjunction with the digital shoreline of Cyprus extracted from the provided DEM. There are ascending and descending observation modes of PALSAR images and differences in backscattering values, therefore, the image calibration is an essential task. Different factors influence backscatter strength signal including satellite ground track, incidence angle, radar polarization, surface roughness and the surface’s dielectric properties (Yingxin & Linlin, 2010). Different objects having the same digital number which may correspond to different backscatter values. Thus, the ALOS scenes were subsequently converted from amplitude data format to normalized radar cross section (σ°) according to Equation 8:

where, DN is Digital Number and CF is a calibration factor (CF = – 83.0 dB).
In SAR image, the speckle noise is one of obstacles to overcome in data processing, so it is necessary to take effective steps to filter the image. Several filter algorithms were tried; the Lee filter was applied to reduce speckle noise. This filter is based on the minimum mean square root (MMSE) and geometric aspects. This is a statistical filter designed to eliminate noise, while still maintaining the quality of pixel points and borders of the image (Hongga et al., 2010).
Atmospheric and geometric corrections were carried out on the GeoEye-1 images. Atmospheric correction is considered to be one of the most complicated techniques since the distributions and intensities of these effects are often inadequately known. Despite the variety of techniques used to estimate the atmospheric effect, the atmospheric correction remains a difficult task in the pre-processing of image data. As it is shown by several studies (Hadjimitsis et al. 2004b, 2010a, 2010b; Agapiou et al., 2011), the darkest pixel (DP) atmospheric correction methodology can easily be applied either by using dark targets located in the image or by conducting in situ measurements.
After the application of atmospheric and geometric corrections to GeoEye-1 images, the research team proceeded in the construction of an overall image mosaic by integrating the two individual images covering the up- and down-stream of the watershed basin (Fig. 9b). For this purpose, a histogram matching technique was applied to the common covered area of the two images in order to secure the radiometric correctness of the final extracted mosaic. Finally, the research team removed the cloud cover from the mosaic image in GIS environment.
GEOEYE-1 IMAGERY CLASSIFICATION AND ALOS PALSAR TEXTURE ANALYSIS
GEOEYE-1 IMAGERY CLASSIFICATION TECHNIQUE
After the application of preprocessing techniques to GeoEye-1 images and the development of an image mosaic, the Maximum Likelihood (ML) algorithm was applied to create a detailed LULC map of the study area. For this reason 7 major classes were defined (Bare rock, Forest, Marl, Soil, Trees, Urban Fabric, Agricultural Areas) (Fig. 11). The statistics of the land use regime of the study area are shown in Table 2. From these statistics, it is clearly seen that the main part of the catchment area is covered by soil and olive trees.
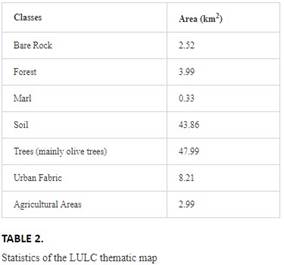
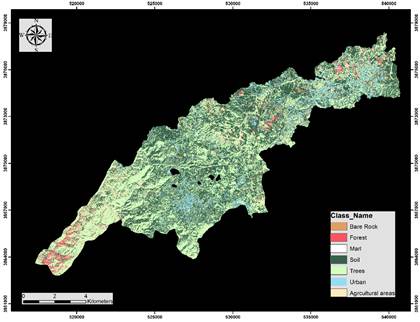
FIGURE 11.
LULC map of the study area after the application of ML classification algorithm to GeoEye-1 mosaic
ALOS PALSAR TEXTURE ANALYSIS
According to Zhang & Zhu et al. (2011) texture is defined as the spatial variation in gray value and is independent of color or luminance. Texture measures smoothness, coarseness and regularity of a region in an image. For the description of texture histograms, gray level co-occurrence matrix (GLCM), local statistics and characteristics of the frequency spectrum are used. The GCLM mainly operates by calculating a matrix that is based on quantifying the difference between the grey levels of neighboring pixels in an image window. The main aim of this matrix is the quantification of the spatial pixel structure within this window. It was initially suggested as a mechanism for extracting texture measures (Haralick et al., 1973).
In the specific study, through the use of ENVI 4.7 software, 7 different statistical indicators of texture such as contrast, angular second moment, homogeneity, entropy, dissimilarity, mean and variance were applied for carrying out the statistical texture analysis of all the typical ground objects. From those textural indicators, multiple RGB composites were constructed to improve the visual monitoring and interpretation of moisture affected areas.
RESULTS AND DISCUSSIONS
As it is clearly seen in Figure 12, in the downward of the catchment area (northeastern part) certain patches were inundated with water. Those patches are clearly observed with the low backscattering values and their corresponding dark pixels. However, in most of the cases the backscattering values were increased mainly because of volume scattering due to the moisture effect in the vegetation and plant cover. Concerning the southwestern part of the watershed where the most forested areas are established, due to the corresponding increase of the moisture after the extreme precipitation event, the backscatter values were generally increased due to the effect of double reflection by water (moisture) and tree trunks. Thus, generally the SAR backscattering intensity in forest areas changes to be higher in cases of inundation events. In addition, in certain areas of the southwestern part of the catchment area where there are more bare rock and soil patterns, the backscattering values were decreased due to the corresponding moisture effect.
The values of radar backscatter coefficient for the different land cover classes as they were extracted from GeoEye-1 images, are tabulated in Table 3. The results were extracted in GIS environment (ArcGIS 10 software) through the use of zonal statistics application. According to Table 3, the backscatter coefficient in most of the classes increased after the precipitation event. The reason for this phenomenon was the overall moisture increase in the area. The backscatter of forest and urban areas was significantly increased (4.57 and 6.67dB) after the precipitation event due to the double reflection phenomenon. On the other hand, in other classes such as soil and bare rock, dB values declined due to water accumulation and the corresponding surface scattering effect. In agricultural areas of low vegetation, such as alfafa or barley crops, the db were slightly increased.
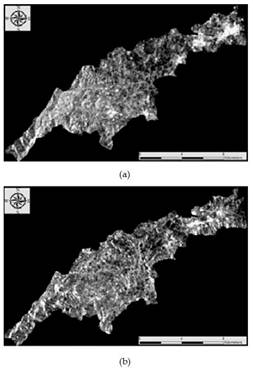
FIGURE 12.
(a) The catchment area before the precipitation event. (b) The catchment area after the precipitation event
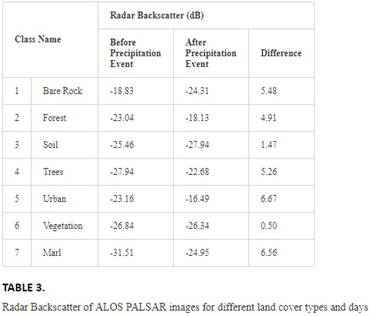
In order to improve image interpretation for water affected areas, several RGB composites were constructed, including microwave and textural bands. The optimum ones improved remarkably the final RGB composites and contributed to the delineation of the moisture affected areas, as shown in Fig. 13. Specifically, in Fig. 13a, the moisture affected areas are indicated in green tones. In Fig. 13b where only texture indicators were used the moisture affected areas are in light cyan color. On the one hand, the combination of speckle reducing Lee filter band and texture indicators in Fig. 13c, resulted in whitish color for flood prone areas. On the other hand, concerning the composite Fig. 13d, the combination of Mean, Variance and Homogeneity bands resulted in a light yellowish color for the moisture affected areas.
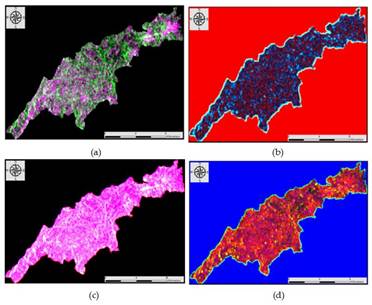
FIGURE 13.
a) RGB composite of the catchment area with the ALOS images before and after the precipitation event (R: Filtered image before precipitation, G: Filtered image after precipitation, B: Filtered image before precipitation – with green colors the areas where backscattering values were increased due to moisture effect are indicated). (b) Texture indicators RGB composite (R: Homogeneity, G: Contrast, B: Dissimilarity) (c). Combination of microwave bands and textural bands (R: Filtered image before precipitation, G: Filtered image after precipitation, B: Mean). (d) Texture indicators RGB composite (R: Mean, G: Variance, B: Homogeneity)
CONCLUSIONS
In this study, ALOS PALSAR imagery data (acquired before and after a certain precipitation event) proved to be useful for evaluating their potential to detect increased land moisture values and to delineate flood prone areas within a catchment area. In the first approach, signal intensity statistics (backscattering values) were extracted to correlate moisture values with certain land cover classes. For this purpose, two high spatial resolution GeoEye-1 images were used to create a LULC map to be used as a reference thematic map.
In addition, texture analysis was employed to ALOS PALSAR images for the detection of flood prone areas. This method is based on the multi-temporal evaluation of the changes that occur between two ALOS PALSAR overpasses before and after the extreme precipitation event. The specific approach aims to highlight the changes and separate this information from unchanged backscatter signals. Moreover, the specific approach is used in order to improve the visual interpretation of SAR images. The visual inspection of filtered ALOS images proved that there is a considerable change in radar backscattering when moisture affects land cover classes. Relative radar backscatter levels sampled in regions of interest and a LULC cover map indicated that different land cover classes yield different backscatter returns in response to moisture/flooding.
The results are useful for examining the potential of ALOS PALSAR images in recording soil moisture regime of an inundated area. However, the research team will continue observation in longer time in case of flooding with the use of radar images. Such information is needed to understand flood mechanism and to better develop water discharge and flood prevention system.
Monitoring urban land cover with the use of satellite remote sensing techniques as a means of flood risk assessment in Cyprus.
INTRODUCTION
This study uses an integrated approach that combines record of urban sprawl, land use and landscape metrics. Specifically, a remote sensing approach is applied to Aster satellite images to analyze and identify patterns of urban changes within the spatial limits of Yialias watershed basin in the island of Cyprus. Moreover, there is an effort to optimize the classification products by combining spectral and texture data to the final.
DATA AND METHODOLOGY
METHODOLOGY
Αn innovative methodology was developed for improving the classification accuracy of Aster images concerning multi-temporal (2000 – 2010) record of urban land cover within the spatial limits of Yialias watershed basin in Cyprus. The phenomenon of spectral similarity of the spectral signatures of urban and marl/chalk formations, identified in the study area, stimulated the calculation of texture measurements in order to improve the traditional classification products derived from spectral bands. Thus, with the use of ENVI 4.7 software 7 indicators of texture information were extracted for the images of 2000 and 2010. These indicators were evaluated for their separability concerning urban and marl / chalk and the optimum ones were used either individually or in combination with spectral bands in order to improve the land use / land cover (LULC) classification accuracy. The Kappa coefficient was used in order to evaluate the reliability of the classified products. In the final stage, the optimum LULC products were incorporated in Fragstats tool in order to record the changes in urban cover structures during the last decade with the use of sophisticated spatial metrics.
DATA
For the purposes of the study, the following satellite and digital spatial data were incorporated:
· A Digital Elevation Model (DEM) of 25m pixel size provided by the Department of Land and Surveys of Cyprus and created with the use of orthorectified stereopairs of airphotos covering the study area.
The acquired ASTER images have a 10 year time interval in order the multi-temporal monitoring of urban sprawl to be guaranteed. For this study, the first three spectral bands were used (VNIR and SWIR) with spatial resolution of 15 m. The exact acquisition dates of the images were: 12 May 2000 and 06 April 2010.
PRE-PROCESSING TECHNIQUES
Geometric corrections were carried out using standard techniques with ground control points and a first order polynomial fit. For this purpose, topographical maps were used to track the position of ground control points in conjunction with the digital shoreline of Cyprus extracted from the provided DEM. in the following, the DN values were converted to radiance values. For both images, the at-satellite radiance values were converted to at–satellite reflectance values. Finally, the darkest pixel atmospheric correction method was applied to every image (Hadjimitisis et al., 2004b). It has been found that atmospheric effects contribute significantly to the classification technique.
IMAGE CLASSIFICATION
In this study, the Iterative Self-Organizing Data Analysis Technique (ISODATA) method was used. The ISODATA algorithm operates as k-means clustering algorithm by merging the clusters if the separation distance in a multispectral feature is less than a value specified by the user and certain rules for splitting a certain cluster into two clusters. Accuracy assessment, which is an integral part of any image classification process, was calculated to estimate the accuracy of different methodologies of land cover classifications. An important statistic generated from the error matrix is the Kappa coefficient that is well suited for accuracy assessment of LULC maps (Vliet, 2009). This statistic takes into account all the values in the matrix and produces an index that indicates the rate of improvement compared to randomly allocating pixels to different classes (Congalton & Green, 2008).
The major issue that this study had to deal with was the similarity of spectral signature response mainly between urban, marl/ chalk and soil features in the Aster images of 2000 and 2010. This problem is clearly denoted in Fig. 14. For this reason different kind of classification methods were used in order to optimize the final results and provide an alternative way of creating efficient LULC cover maps.
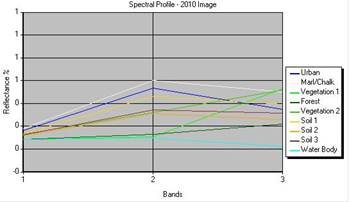
FIGURE 14.
Spectral response curve of typical ground objects
MULTISPECTRAL IMAGE CLASSIFICATION
The pixel-based classification is considered to be the most classic way of classifying satellite imagery. For this reason, the first three bands of Aster image were used covering a spectral range from visible to near infrared part of spectrum. This process was accomplished in order to form a standard of comparison with the other classification products such as those of texture or combination of texture and spectral bands. After proceeding with evaluation accuracy, it was resulted that the Kappa coefficient for image acquired for 2000 was 0.684 and for 2010 was 0.695. These accuracies can be described as moderate and were ascribed to urban and marl/chalk spectral conflict.
TEXTURE CLASSIFICATION
According to Zhang & Zhu (2011), texture is defined as the spatial variation in gray value and is independent of color or luminance. Texture measures smoothness, coarseness and regularity of a region in an image (Gonzalez & Woods, 1992). Concerning satellite digital imagery texture quantifies the way two neighboring pixels relate each other within a small window centered on one of the pixels. It is generally used to describe the visual homogeneity of images and is considered to be a common intrinsic property of all ground objects. For the description of texture histograms, gray level co-occurrence matrix (GLCM), local statistics and characteristics of the frequency spectrum are used. The GCLM mainly operates by calculating a matrix that is based on quantifying the difference between the grey levels of neighboring pixels in an image window. The main aim of this matrix is the quantification of the spatial pixel structure within this window. It was initially suggested as a mechanism for extracting texture measures (Haralick et al., 1973).
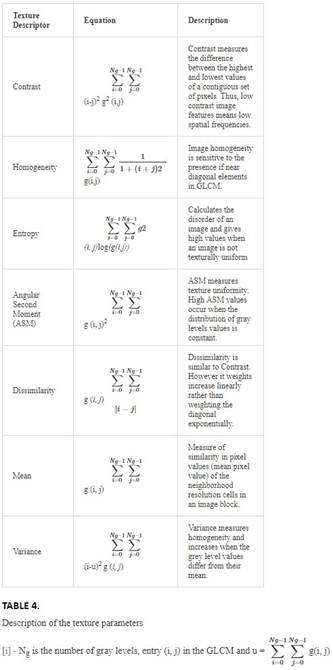
Initially, principal component analysis was applied to both satellite images in order to extract the first principal component from each image which would subsequently be used for texture analysis. Thus, the first component of the two images was imported in ENVI 4.7 software and 7 different statistical indicators of texture such as contrast, angular second moment, homogeneity, entropy, dissimilarity, mean and variance were used for carrying out the statistical texture analysis of all the typical ground objects (Tables 4, 5 and 6).
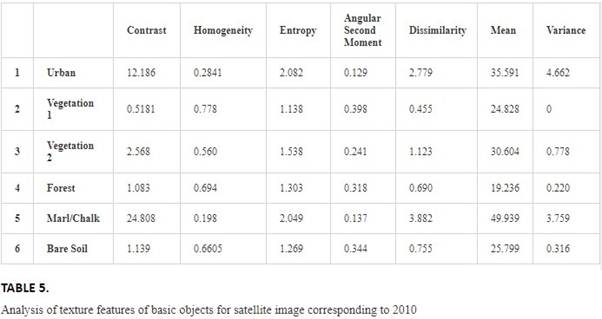
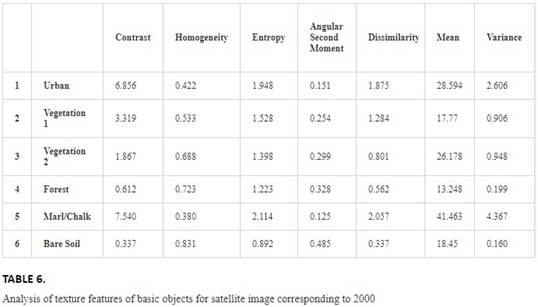
It is clearly shown in Table 5 that marl formations and urban classes which cannot be differentiated (based on spectral features) vary in the means of contrast, homogeneity, dissimilarity and mean texture regarding the image corresponding to 2010 (Fig. 14). Concerning the texture bands of 2000 (Table 6)the greatest differences in values between marl and urban classes are indicated at mean and variance texture classes.
Texture-based classification methodologies give the opportunity to end users to extend the traditional-based classifiers by incorporating the texture bands into the multispectral bands, in order to coalesce the spectral and spatial information in the final product. The ISODATA algorithm was applied to different texture products. Specifically, the algorithm was applied to the multiband texture images of 2000 and 2010 and to the PCA products (three first components) of 2000 and 2010 with corresponding Kappa coefficients of 0.694, 0.685, 0.710, 0.715 and 0.723.
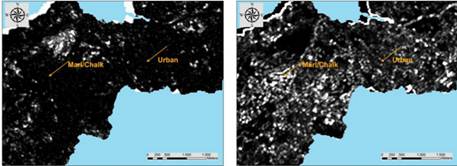
FIGURE 15.
Urban and marl/chalk features as indicated in Angular Second Moment texture indicator (left). Urban and marl/chalk features as indicated in Contrast texture indicator (right)
COMBINED SPECTRAL AND TEXTURE METHODOLOGY
The combined use of spectral and texture methodology function by combining spectral and texture bands (either original bands or PCA components) and creating a final integrated image. For this study, the following two combinations were accomplished and the ISODATA classifier was applied to them:
· Use of all multispectral and texture bands
· Use of all multispectral bands and the first three components after the application of PCA to texture bands.
The overall accuracy of the methodology was considered as promising compared to the results of the previous classification products derived from individual either spectral or texture bands. Specifically, the Kappa coefficient values for the 1st category of combined classification for 2000 and 2010 was 0.702 and 0.732, respectively. In addition, the Kappa coefficient values for the second category were 0.765 and 0.775 concerning 2000 and 2010 images. These results led the research team to select these two final LULC cover maps concerning the period 2000 and 2010 for applying spatial landscape metrics.
LANDSCAPE METRICS
Spatial landscape metrics are used in sustainable landscape planning and analysis of urban land use change (Botequilha et al., 2002). These metrics typically measure spatial configuration of landscapes, and can be used to enhance the understanding of relationships between spatial patterns and spatial processes (Herold et al., 2005). In this study, the FRAGSTATS tool was used in order to measure and analyze the diachronic changes of LULC regime of the study area and record the urban sprawl phenomenon within the watershed. Specifically, seven spatial individual metrics were used for analyzing urban land cover changes and these were (Edge Density, Largest Patch Index, Class Area, Number of Patches, Area weighted mean patch fractal dimension, Euclidean nearest neighbor distance and Contagion) (Table 7).
As investigated by O’Neil et al. (1988) due to correlation and overlap between landscape metrics, it is not necessary to calculate all landscape metrics. The specific metrics were selected because of their simplicity and effectiveness in depicting urban forms evolution (Alberti & Waddel, 2002; Herold et al., 2002). It was found that there was an increase in built up areas during the period 2000 to 2010. The number of patches used in landscape analysis indicate the aggregation or disaggregation in the landscape. The considerable increase of the specific index during the time span 2000 – 2010 suggests urbanization in the study area characterized by dispersion. Moreover, a development of a number of isolated and fragmented built up areas occurred at the end of this period. Regarding largest patch index, the small increase between 2000 and 2010 indicates a corresponding small urban core increase. The increased urbanization rate is characterized by the appearance of new, dispersed settlements.
TABLE 7.
Properties of spatial metrics used in this study
Thus, the increase of edge density value by indicates an increase in the total length of the edge of the urban patches due to urban land use fragmentation. This finding is also enhanced by the increase in weighted mean patch fractal dimension value indicating the urban sprawl phenomenon in the study area. Moreover, the fractal shape dimension value was always slightly higher than 1, indicating a moderate shape complexity. In addition, the decrease in Euclidean Nearest Neighbor Distance metric between 2000 and 2010 denoted a reduction in the distance between the built-up patches, suggesting coalescence (Table 8).
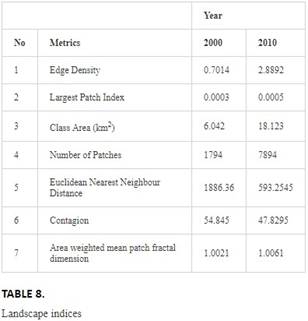
However, it is important to mention that the landscape metrics results can be used as general indicators and do not provide the users with absolute answers.
RESULTS
The impacts of changes in land use patterns on hydrology due to extensive urbanization in the spatial limits of watershed is a critical issue in water resource management and watershed land use planning. Land use and land cover maps of the study area for the years 2000 and 2010 were obtained using spectral bands, texture bands or combination of both of them. The major motivation for the use of alternative classification methodologies was the existence of similar spectral signatures for urban and marl/chalk geologic formations located in the study area. These methodologies were evaluated for their accuracy and the optimum classification products were selected in order to be used to the research of urban land use regime evolution during the last decade. In both cases (2000 and 2010) the combination of three spectral bands with the first three principal components extracted from texture bands led to more accurate and reliable results. In the next stage, landscape spatial metrics were used to measure the urban sprawl phenomenon in the study area and its changes through time. Specifically, seven metrics were applied to the two final classified images. The results from the vast majority of the metrics, besides Euclidean distance measurement, denoted a steady dispersion of urban settlements within the area of watershed. Although there was not a significant total urban area increase during this period, a considerable urban sprawl phenomenon was recorded.
This study denoted that spatial measures, such as texture, can play an important role in the analysis of satellite imagery. The overall improvement of classification accuracy products derived from images of medium spatial resolution such as those of Aster highlights the potential of use of texture bands in combination with multispectral imagery. Moreover, the urban sprawl phenomenon was recorded in detail with the use of landscape metrics emphasizing to the flood inundation danger in an already flood prone watershed basin such as Yialias. The research team will continue the specific research by incorporating images of higher spatial resolution to the classification model.
Overall conclusions
This study revealed that the integrated use of satellite remote sensing and GIS technology can contribute substantially to the sustainable management of a watershed basin. Interpretation of multi-spectral satellite sensor data proved to be of great help in the development of updated LULC maps and record of the LULC regime and urban sprawl phenomenon in a catchment area. Moreover, a soil erosion model such as RUSLE was found to be efficiently applied at basin scale with quite modest data requirements in a Mediterranean environment. The RUSLE model provides the end users with reliable quantitative and spatial information concerning soil erosion and erosion risk in general. Following, the results denoted the potential of Radar imagery in recording soil moisture regime of an inundated area as well its potential to improve classification accuracy.
The overall results pointed out the substantial contribution of satellite remote sensing to the sustainable management of a catchment area.
Comments are closed